NEW Updates to the Matrix Visual in Power BI | May 2024 Update
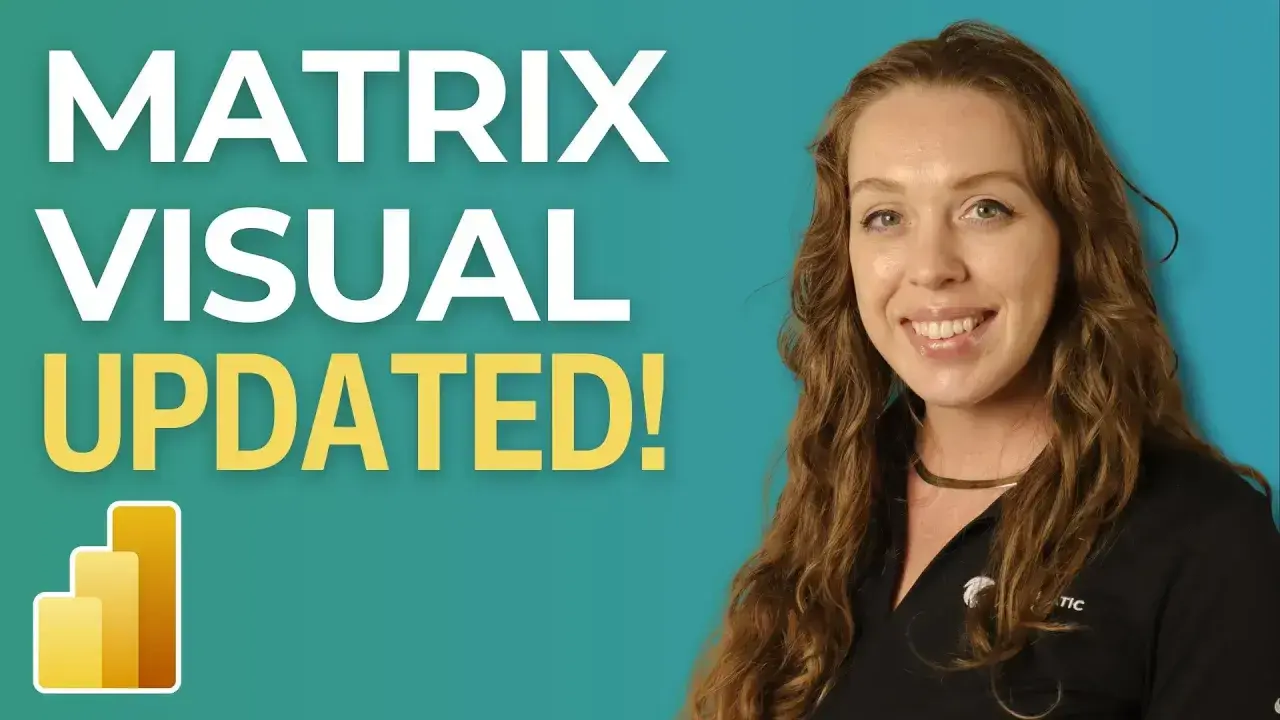
Start with the FREE community plan and get your lifetime access to 20+ courses. Get Instant Access Now!
Need help? Talk to an expert: (904) 638-5743
Private Training
Customized training to master new skills and grow your business.
On-Demand Learning
Beginner to advanced classes taught by Microsoft MVPs and Authors.
Bootcamps
In-depth boot camps take you from a novice to mastery in less than a week.
Season Learning Pass
Get access to our very best training offerings for successful up-skilling.
Stream Pro Plus
Combine On-Demand Learning platform with face-to-face Virtual Mentoring.
Certification Training
Prepare and ace your next certification with CertXP.
Private Training
Cheat Sheets
Quick references for when you need a little guidance.
Nerd Guides
Summaries developed in conjunction with our Learn with the Nerds sessions.
Downloads
Digital goodies - code samples, student files, and other must have files.
Blog
Stay up-to-date on all things Power BI, Power Apps, Microsoft 365 and Azure.
Community Discord Server
Start here for technology questions to get answers from the community.
Career Guides
Breaking into the field? Let these guides help get you started with a plan.
Affiliate Program
Earn money by driving sales through the Pragmatic Works' Training Affiliate Program.
Reseller Partner
It's time to address your client's training needs.
Foundation
Learn how to get into IT with free training and mentorship.
Management Team
Discover the faces behind our success: Meet our dedicated team
Contact Us
How can we help? Connect with Our Team Today!
FAQs
Find all the information you’re looking for. We’re happy to help.
free events
A free virtual series bringing thousands of tech professionals together for a few hours covering an array of data analytics and cloud topics.